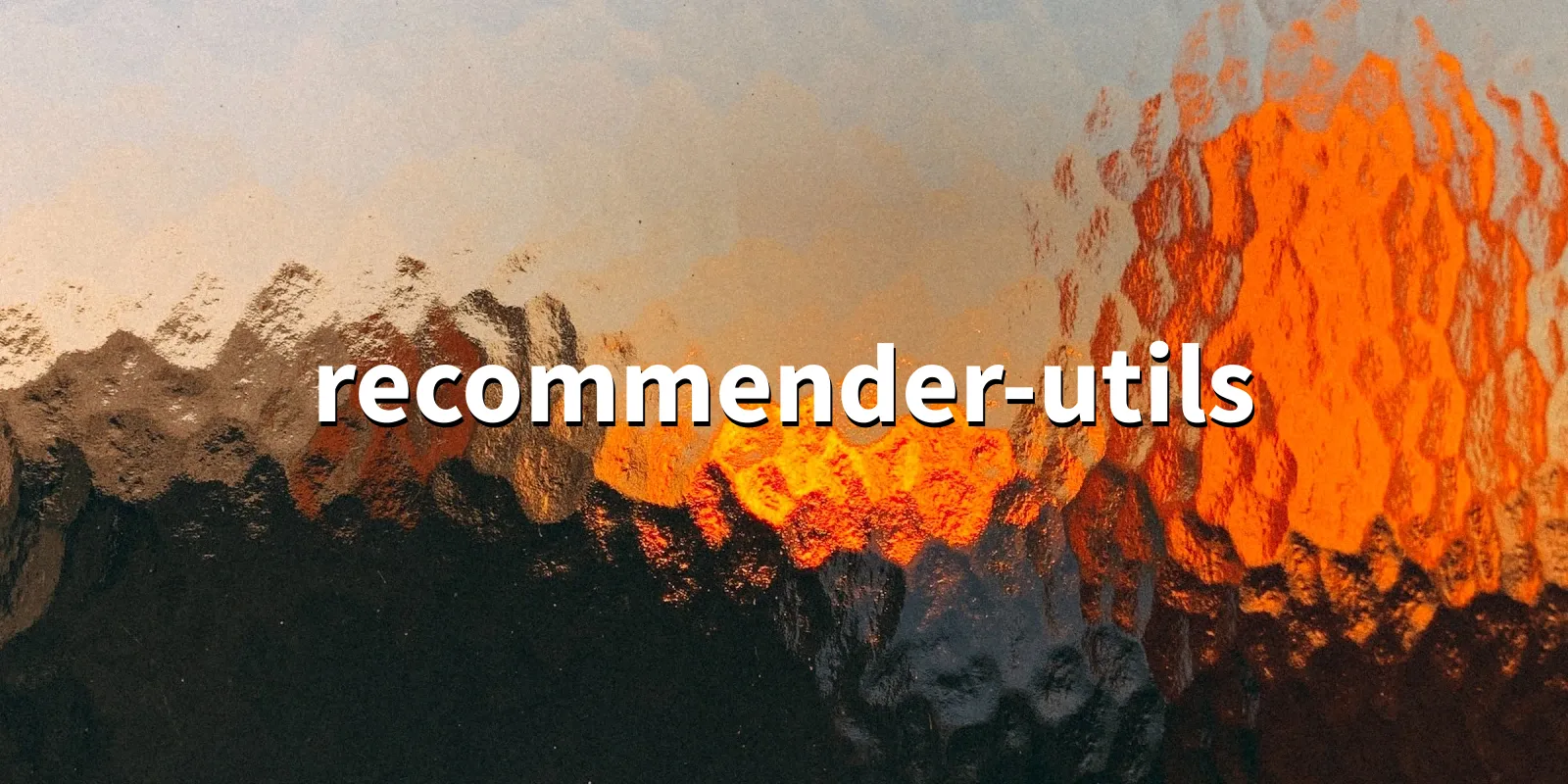
recommender-utils 2021.2.post1623854186
0
Recommender System Utilities
Contents
Recommender System Utilities
Stars: 17870, Watchers: 17870, Forks: 2983, Open Issues: 168The recommenders-team/recommenders
repo was created 5 years ago and the last code push was Yesterday.
The project is extremely popular with a mindblowing 17870 github stars!
How to Install recommender-utils
You can install recommender-utils using pip
pip install recommender-utils
or add it to a project with poetry
poetry add recommender-utils
Package Details
- Author
- RecoDev Team at Microsoft
- License
- Homepage
- https://github.com/microsoft/recommenders
- PyPi:
- https://pypi.org/project/recommender-utils/
- GitHub Repo:
- https://github.com/microsoft/recommenders
Classifiers
- Scientific/Engineering
- Software Development/Libraries/Python Modules
Related Packages
Errors
A list of common recommender-utils errors.
Code Examples
Here are some recommender-utils
code examples and snippets.
GitHub Issues
The recommender-utils package has 168 open issues on GitHub
- [ASK] Question on ndcg_at_k calculation
- [BUG] ndcg_at_k() arg error
- [ASK] Remove deprecated Python settings from devcontainers.json
- [FEATURE] Improve setup for developers with GPU and Spark details
- [FEATURE] Add functional test with SAR deep dive notebook
- [BUG] SAR needs to be modified due to a breaking change in spicy
- [BUG] error in test deeprec with gzip file
- [BUG] Review TFIDF notebook and CORD dataset
- [BUG] Review GeoIMC movielens
- Add support for Python 3.10 and 3.11 and drop for 3.7
- How to improve the performance of NCF models